Research
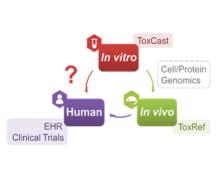
Developing a new therapeutic today costs in excess of $2 billion and can take more than a decade before making it to the patient. This cost is largely driven by high rates of attrition of drug candidates, including those advancing to clinical trials. A significant cause of this attrition is unpredicted toxicity. We are addressing the issue of high attrition during drug discovery by developing predictive methods for identifying drugs with a high risk of causing tissue. Specifically, we are building models for drug induced liver injury (DILI), which has been identified as one of the primary reasons for clinical trial failure for compounds across drug classes and disease indications.
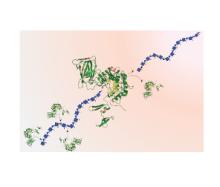
We are using biocatalysis in production of animal-free glycosaminoglycans (GAGs), including the world’s highest volume anticoagulant, heparin. We are also using biocatalysis in energy-related research, with a focus on designing metabolic pathways using cell-free metabolic pathway engineering coupled with electrochemical bioreactors and use of enzymes to regenerate NAD(P)+ from inhibitory NAD(P)H isomers. Finally, we are using cell-free pathway engineering to generate various (bio)chemicals with high efficiency.
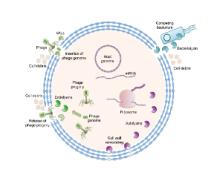
We are exploiting nature’s biocatalytic defense mechanisms to combat microbial and viral infections, overcome bacterial resistance mechanisms, and applying our approach to surfaces within common infrastructures, including hospitals, schools, food processing facilities, etc. Our approach involves two classes of enzymes; peptidoglycan hydrolases and oxidative biocatalysts. The former are lytic enzymes, which are extremely selective and do not require reagents apart from water to act. The oxidative enzymes, including oxidases, peroxidases and perhydrolases, are generally nonselective and require addition of reagents to catalyze their microbicidal activity. In both classes, these enzymes can be used in their soluble form or embedded into materials that can be used to coat surfaces and kill bacteria on contact. We are also developing various routes to novel antivirals, including broad-based coronavirus inactivation based on perhydrolases and sulfated polysaccharides.
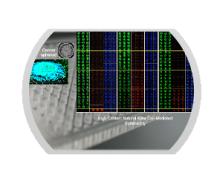
We are developing new cell engineering and biomolecular tools that can expand the repertoire of cellular control, accelerate drug discovery, control stem cell fate and function, develop 3D and spheroid cell culture platforms, and identify potential for immune escape from vaccines.
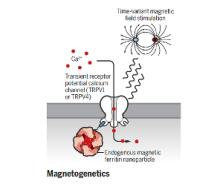
The brain is a network, and as we learn more about the pathophysiology of neurologic diseases, it is increasing clear that they are not isolated to a specific brain region but emerge from a dysfunction in brain networks. Many diseases are also a direct or indirect result of inflammatory events both in the brain and systemic. Indeed, epilepsy, Alzheimer's disease, Parkinson’s disease, neuropathic pain and depression all exhibit loss of neurons and reduced neuronal density as well as altered connectivity of key regions, and in many cases, the hallmark of inflammation is coincident with such neuronal death. As we begin to identify some of the circuits common to pathophysiology of many of these diseases, we can take advantage of new, minimally invasive, highly targeted interventions, such as neuromodulation, to help treat patients with debilitating symptoms but no cure. We are focusing on two quite distinct neuromodulatory pathways: (1) magnetogenetics and (2) control of circadian rhythms, both that can address potential therapeutic modalities in the future. However, our studies to date remain focused on gaining a mechanistic understanding of these two areas of neuromodulation.