Developing a new therapeutic today costs in excess of $2 billion and can take more than a decade before making it to the patient. This cost is largely driven by high rates of attrition of drug candidates, including those advancing to clinical trials. A significant cause of this attrition is unpredicted toxicity. We are addressing the issue of high attrition during drug discovery by developing predictive methods for identifying drugs with a high risk of causing tissue injury [Fraser et al. Chem. Res. Toxicol. 31, 412-430 (2018)]. Specifically, we are building models for drug induced liver injury (DILI), which has been identified as one of the primary reasons for clinical trial failure for compounds across drug classes and disease indications. Our approach takes advantage of machine learning tools to mine and analyze biomedical data across a range of structured and unstructured data warehouses (Figure 1). This work builds on our high-throughput in vitro platform for screening drug toxicity and leverages the high-performance computing capabilities through the Rensselaer Center for Computational Innovation (CCI) and the Cognitive and Immersive Systems Lab (CISL).
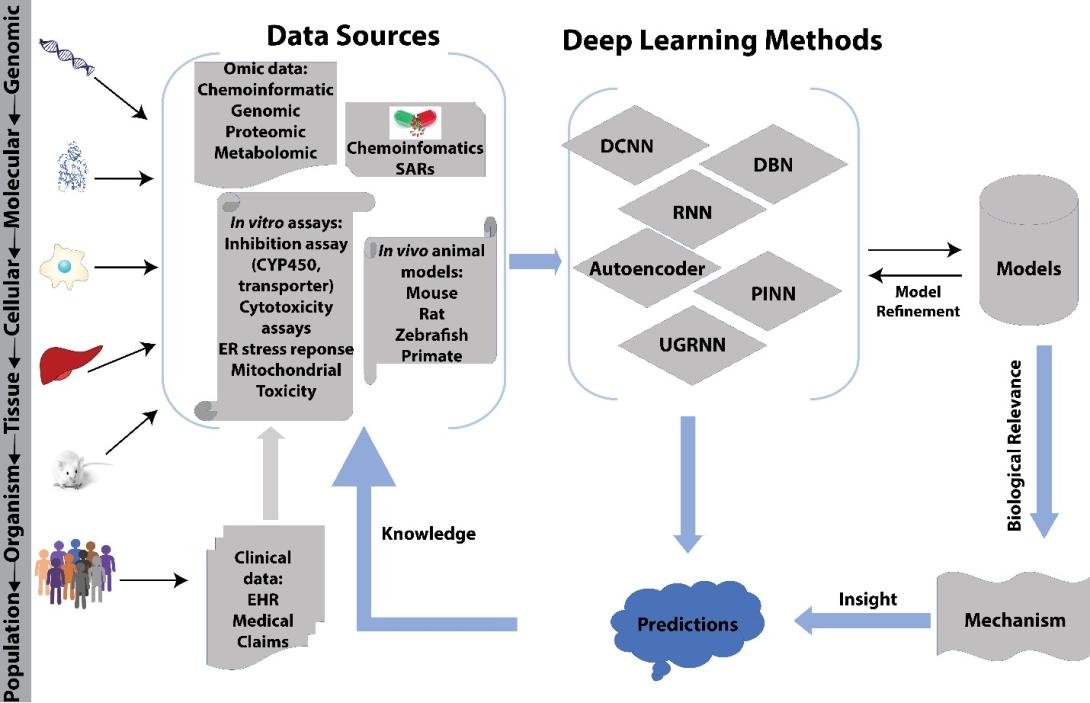
Figure 1. Computational/experimental paradigm for drug discovery, which includes cognitive systems for exploring new dimensions of biomedical information [Fraser et al. Chem. Res. Toxicol. 31, 412-430 (2018)].
Existing drug toxicity data in literature, while limited, is not without value, and has led to numerous in silico models being applied to predict toxicities of drugs before clinical phase trials. However, there remains relatively poor concordance between in vitro / in vivo / human toxicity as well as a large amount of chemical, biological, and mechanistic data that has been applied to predict outcomes of varying granularity and relevance to toxicity in humans. We are addressing a variety of machine learning models that encompass in vitro activity at the cellular level and very broad toxic/non-toxic predictions in an entire animal or human (Figure 2). In particular, we are using a deep learning framework for modeling in vitro, in vivo and human toxicity data simultaneously. [Sharma et al. Sci. Rep. 13, 4908, 2023]. With pre-trained Simplified Molecular Input Line Entry System (SMILES) embeddings as input, the multi-task model yielded improved or on par clinical toxicity predictions to current baseline and state-of-art molecular graph-based models. Unlike graph neural nets, our framework takes advantage of reduced inference costs from language models when using pre-trained SMILES embeddings. Our results strongly suggest that there is a minimal relative importance of in vivo data for predicting clinical toxicity in particular when unsupervised pre-trained SMILES embeddings were used as an input to multi-task models; thus, providing possible guidance on what aspects of animal data cannot be considered in predicting toxicity. To our knowledge, this is the first work to explain with both present and absent substructures predictions of clinical and in vivo toxicity.
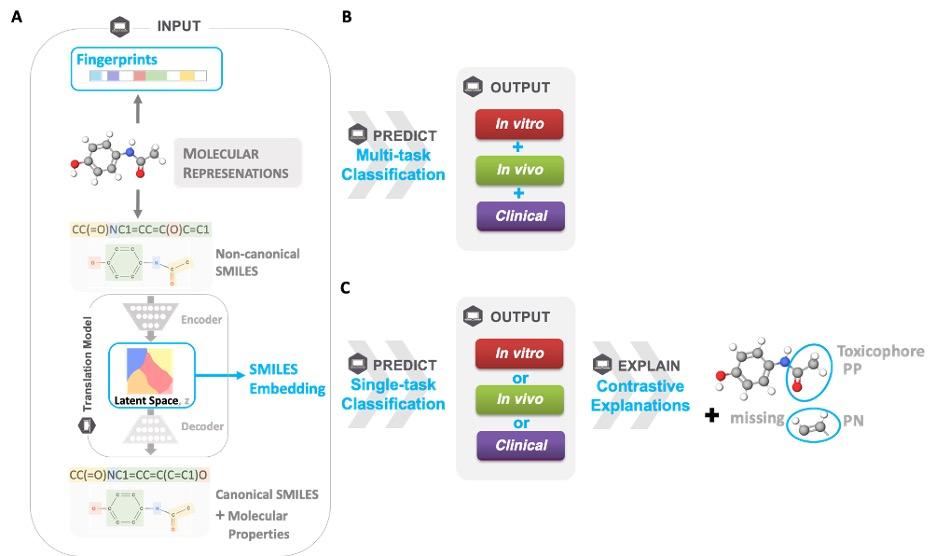
Figure 2. Predicting toxicity in humans, Framework adopted in this study for explainable, single-task, and multi-task prediction of in vitro, in vivo, and clinical. A) Given an input of different molecular representations, fingerprints and latent space SMILES embeddings, B) a multi-task classification model predicts whether a molecule is toxic or not for in vitro, in vivo, and clinical endpoints. Furthermore, the Contrastive Explanation method explains C) predictions from single-task models trained on fingerprints for the same endpoints. The method pinpoints minimal and necessary chemical substructures that are either present (pertinent positive, PP) or absent (pertinent negative, PN) for a specific prediction.
Current Collaborators:
Payel Das – IBM Research
James Hendler – Rensselaer Polytechnic Institute